- May 6, 2025
How Generative AI Is Radically Transforming Data Engineering
Data engineering no longer pertains just to transferring data from point A to B. It now enters a new era, one that is strictly dependent on automation, intelligence, and flexibility.
Generative AI lies at the heart of this evolution, presenting functionalities which seemed futuristic a few years ago in enterprise workflows in the present.
The fast emergence of data sources, real-time demands, and AI-driven analytics have put enormous pressure on what once used to be called ‘traditional’ data practices.
Businesses need smarter, more dexterous systems now. From automating documentations to designing complex pipelines through simple prompts, here is how GenAI changes the way a data engineer builds, monitors, and optimizes its systems.
Come, let’s get started!!
What Is Generative AI in the Context of Data Engineering?
- Quick GenAI Refresher
Generative AI has models like GPT, Claude, and open-source LLMs that have trained on a large data corpus. These models are capable of generating text, code, logic, and insights.
- Data Systems Intersection
GenAI is now integrating with ETL tools, data catalogs, and orchestration layers, providing unprecedented levels of automation and intelligent management for the data flows, transformations, and quality checks.
It is the human that instructed and programmed each traditional data pipeline with rules, transformations, and manual configuration.
With generative AI in data pipelines, engineers can now create, modify, and troubleshoot data flows using natural language.
Prompt-driven tools can automatically write transformation logic, validate data, and generate test cases, thus bringing down the development cycle from several weeks to just a few days.
Imagine an entire data ingestion and transformation mechanism being created by acting upon a description in plain English. This is the magic of GenAI-intelligent, adaptive, and low code pipelines.
AI-Driven Transformation of Data Architecture
AI is redefining the entire conceptual framework within which data architectures traditionally operate into the adoption of existing generic layered architectures, it is now possible to build flexible, context-aware systems according to GenAI, among other things:
- Automatic generated schema based on sample data or prompts
- Intelligent observability has helped detect bottlenecks or anomalies
- Flexible AI-augmented metadata for more intelligent discoverability.
It will transform the initial rigid architecture into a responsive, scalable, and self-healing architecture engineered for real-time decision-making.
GenAI for Data Governance - More Intelligent, Context-Aware, and Scalable
Traditionally, governance is an impediment because it is labor-intensive. The new GenAI for data governance changes this; policy formulation, access and compliance, are no longer static:
- Automatically classify sensitive data, mask, or encrypt
- Generate data lineage diagrams without human intervention
- Context-aware tags ensure associated usage metadata are available when required
This creates a future adaptation of AI-facilitated governance as data flow patterns change, making them more secure and productive.
The Real-World GenAI Applications in Data Engineering
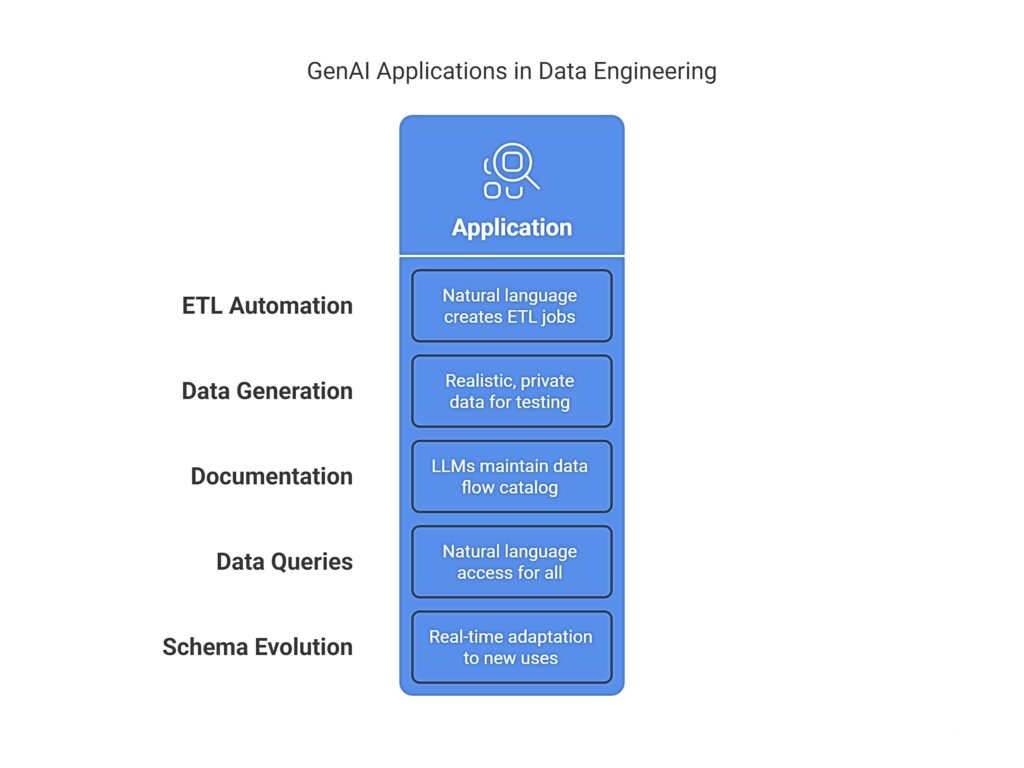
GenAI is being actively utilized in companies clustered in different industries as seen in some of the top GenAI use cases applied in real life. These include:
- AI-powered ETL automation: Use natural language to create entire jobs for ETL.
- Synthetic data generation: Privacy-compliant, realistic data for tests & model training.
- Documentation and lineage tracking: LLMs will maintain a complete catalog of data flows.
- Natural language queries: Democratizing access to data for non-technical users.
- Dynamic scheme evolution: Real time adaptation based on new use cases.
The above instances contribute to reduced manual input by increasing agility and opening new perspectives regarding data consumption and interpretation.
Data Quality in the Age of AI-Powered Validation
The maintenance of high-quality data is an unending task. The GenAI models for AI-augmented data quality systems:
- Provide detection of outliers and anomalies from crawler massive datasets.
- Suggest remedies or transform fixes by themselves.
- Bring in explainability to failed validations.
This provides teams the power to manage quality proactively instead of reactively encountering problems downstream.
Key Benefits of Using Generative AI in Data Engineering
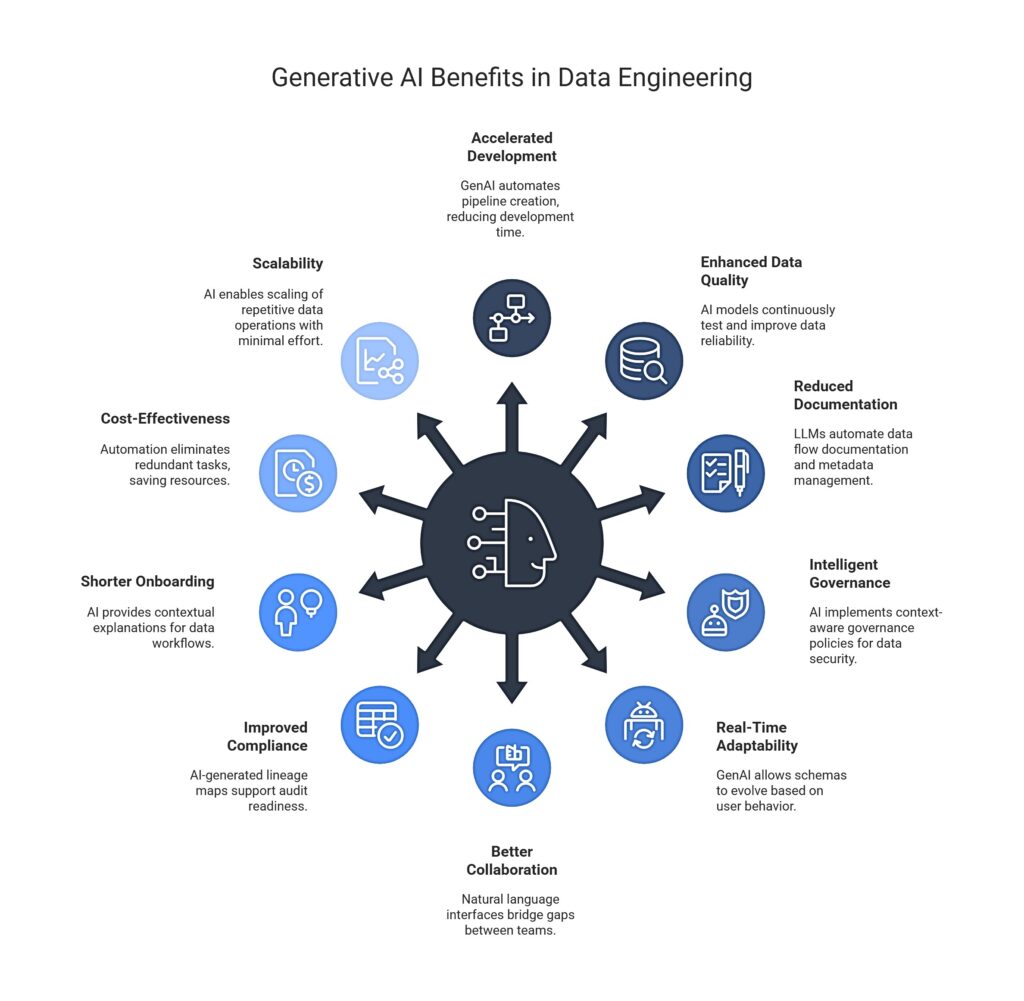
The new Generative AI, intelligent automation, collaborative tools, and greater efficiency are changing the face of data engineering:
- Accelerated Development of Data Pipelines: GenAI generates transformation logic and builds pipeline components automatically, significantly reducing development time.
- Enhanced Data Quality with AI-Driven Testing: The AI models test datasets, detect anomalies, and improve them on an ongoing basis, increasing data reliability and trustworthiness.
- Less Documentation and Metadata Management by Hand: LLMs document the data flow, generate the technical metadata, and maintain lineage, one of the boring chores.
- Intelligent Governance with Context-Aware AI: With GenAI, governance policies are implemented in context of data ensuring smarter security and classification on auto-mode.
- Real-Time Schema Adaptability: GenAI allows schemas to evolve in real-time based upon user behavior or changes to data inputs, thus giving agility to the system.
- Better Collaboration through Natural Language Interfaces: Teams can verify, update, or monitor pipelines in plain English, bridging gaps between data engineers and business users.
- Improved Compliance with AI-Annotated Lineage: Clear AI-generated lineage maps track data origin, transformation, and usage, supporting audit readiness.
- Shorter Onboarding through Auto-Explainers for Pipelines: GenAI gives contextual explanations for complicated data workflows, helping to ramp up new team members quicker.
- Cost-Effectiveness through Eliminating Redundant Engineering Tasks: Automating repeat transformation and validation tasks saves time and resources.
- Scalability of Repetitive DataOps Procedures: GenAI provides teams with the ability to scale monitoring, validation, and transformation, with minimum effort.
Risks, Challenges & Limitations
If GenAI is perceived as a disruptive technology, it brings forth challenges that must be dealt with in a systematic way:
- Pipeline Logic Hallucinations or Errors: LLMs could generate bad code that seems correct. Validation layers, therefore, have to be in place.
- Data Privacy and Model Complaints: GenAI use would become a problem with sensitive datasets if encryption, access, and data masking were not meticulously put in place.
- Heavy-Lifting for Legacy Integrations: Integrating GenAI into legacy systems may involve changes in architecture, thereby adding to the complexity of initial implementation.
- Human Intervention Is Needed: Although process replacement provides for automation, much more needs to be considered by the human mind in instances of correctness, security, and business relevance.
- Lack of Explainability of GenAI Decisions: There are instances when GenAI is not very good at explaining what it does, which makes justifying its decisions or meeting compliance targets that little bit tougher.
Conclusion
Generative AI is rewriting the future of data engineering. What was once manual, rule-based, and inflexible is being molded into an intelligent, autonomous, and adaptive ecosystem.
Whereas GenAI provides ways to enhance pipeline building and maintenance, it also improves governance and compliance, collaboration, and scalability.
It is time to explore, embrace, and experiment. Organizations starting today with GenAI integration will become the frontrunners in data innovation.
Happy Learning!!
FAQs
1. How is generative AI being used in real-world data engineering projects?
GenAI is automating data transformation logic, pipeline generation, documentation, schema evolution, and even natural language querying, which significantly reduces manual work.
2. What does a GenAI-powered data architecture look like?
Automation of every layer will be powered by intelligence i.e., intake using AI, dynamic schemas, automated lineage, self-validating pipelines, and natural language interfaces integrated into the data workflow.
3. Will generative AI replace traditional ETL tools?
Rather, GenAI has been growing ETL by automating and accelerating operations and will leave the original ETL tools in use, especially in cases of complexities or legacy.
4. What changes should companies make to prepare for GenAI in data engineering?
These involve investments in AI-enabled data platforms, upskilling teams on prompt engineering and AI literacy, and modernizing data infrastructures to have a flexible, compliant, and observability architecture.