- May 13, 2025
SMBs are now facing a crucial choice! Especially on how to manage, access, and leverage data for overall business empowerment. Emerging with the unprecedented data generated by the day from various teams and platforms, the concepts of Data Mesh and Data Fabric have comparatively arisen in recent times.
With each heralding unparalleled scalability, agility, and intelligence with respect to data handling, they share contrasting undercurrents. It is, however, imperative to note that understanding the difference between Data Mesh and Data Fabric becomes a core aspect of a good data strategy selection with the backing of organizational goals.
Being an SMB, there is a need to find better ways of utilizing data to remain competitive. Traditional ones, such as centralized data lakes, are now quite frankly buckling under demand for real-time activities, dispersed worldwide teams, and accountability.
By contrast, traditional data systems leaning toward centralized control are practically archaic in the view of modern organizations that are in fact looking for new age decentralized and dynamic systems.
This blog will facilitate your decision-making and influence your teams’ ability to access insights, innovate products, and drive ROI. For SMBs, the slightest chance of going for the wrong warehouse model could mean sabotaging efforts or staying put.
Quick Overview: What Are Data Mesh and Data Fabric?
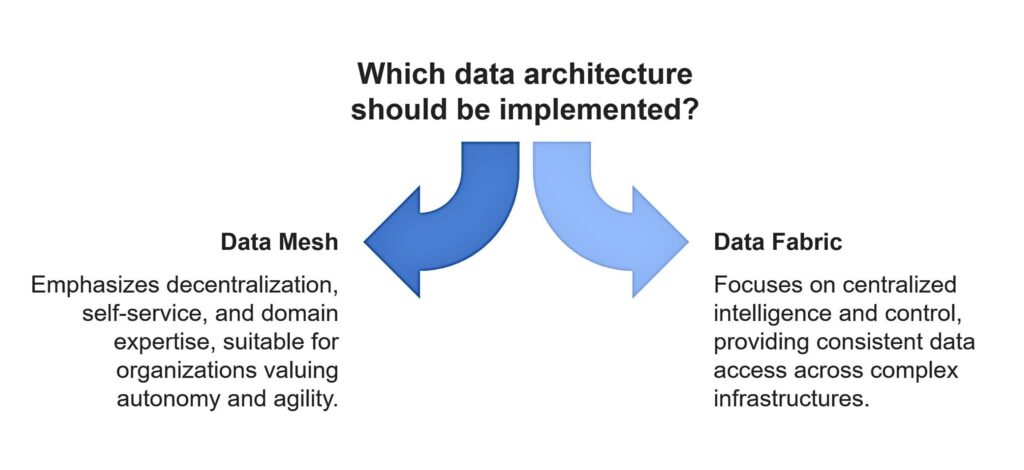
At first glance, Data Mesh and Data Fabric may appear quite similar, as both aim to simplify data access and accelerate any decision-making. However, there is a vast difference in their approach and intention.
Data Mesh is an architectural paradigm that treats data as a product, thereby maintaining an individual’s ownership from business domains like marketing, sales, or HR. The other way around, Data Fabric offers a panoply of technologies and services working across different environments-cloud, on-premises, or hybrid-to automate data integrations, discovery, governance, and delivery.
Intelligent metadata management does play a very crucial role in providing consistent and real-time access to data across the enterprise. The philosophies that govern these two systems vary greatly. While the Data Mesh places importance on decentralization, self-service, and domain expertise, it applies well in organizations where autonomy and agility are comparatively maintained.
On the contrary, Data Fabric is about centralized intelligence and control, providing consistent data access across complex infrastructures. In terms of technology, Data Mesh usually employs APIs, event streaming platforms like Kafka, decentralized governance frameworks, and cloud-native tools to support domain ownership and flexibility.
While Data Fabric relies heavily on AI/ML-driven metadata management, knowledge graphs, data virtualization, and sophisticated integration tools such as Informatica or Talend, to create a connected and intelligent data layer.
Key Differences Between Data Mesh and Data Fabric
Benefits of Each Approach
With data mesh, here are some advantages:
- Scalability across teams and domains: Allows each team to manage and grow their own data products internally.
- Stronger Ownership and Accountability: Invincible in imposing shared responsibility and pinpoint data ownership with domain experts.
- Better fit for agile DevOps-style cultures: Suits distributed teams, rapid iteration models, and golden standard patterns today in more modern DevOps environments.
- Freedom of technology choice: Allows domains to consume the suitable tools and platforms useful for their specific use cases and workflows.
- Encourages local-level innovation: Opens lateral access to localized experimentation in innovation rather than being bottlenecked by centralized control.
Feature | Data Mesh | Data Fabric |
---|---|---|
Architecture | Domain-driven and decentralized, each business unit manages its data. | Centralized and integrated; focuses on creating a unified data access layer. |
Data Ownership | Ownership is distributed across teams or domains for better accountability. | Data is centrally managed with automated orchestration and metadata intelligence. |
Tech Stack | Open, flexible, and polyglot; uses APIs, streaming, and microservices. | Platform-centric with reliance on AI/ML, metadata tools, and enterprise integration. |
Governance | A federated model with domain-level governance frameworks and standards. | Centralized governance is driven by policies, automation, and metadata management. |
Use Case Fit | Ideal for complex, fast-moving organizations with domain-specific needs. | Best for enterprises needing consistency, real-time access, and unified data visibility. |

However, some benefits associated with data fabric networks include:
- Full visibility across the enterprise: Transparency and decision-making enhanced by a complete view of data across all systems.
- Improved accessibility for AI and ML analytics: Seamlessly links high-quality data sources necessary for machine learning and sophisticated analytics.
- Real-time Integration and delivery of data: Automation provides real-time data movement and synchronization with instantaneous data flow and timely access.
- Less duplication and inconsistency in data: Integration and governance at one center can resolve the problem of redundancy.
- Well-automated governance and compliance: Supported by built-in automation and metadata intelligence, a consistent set of data policies are applied, and compliance is assured.
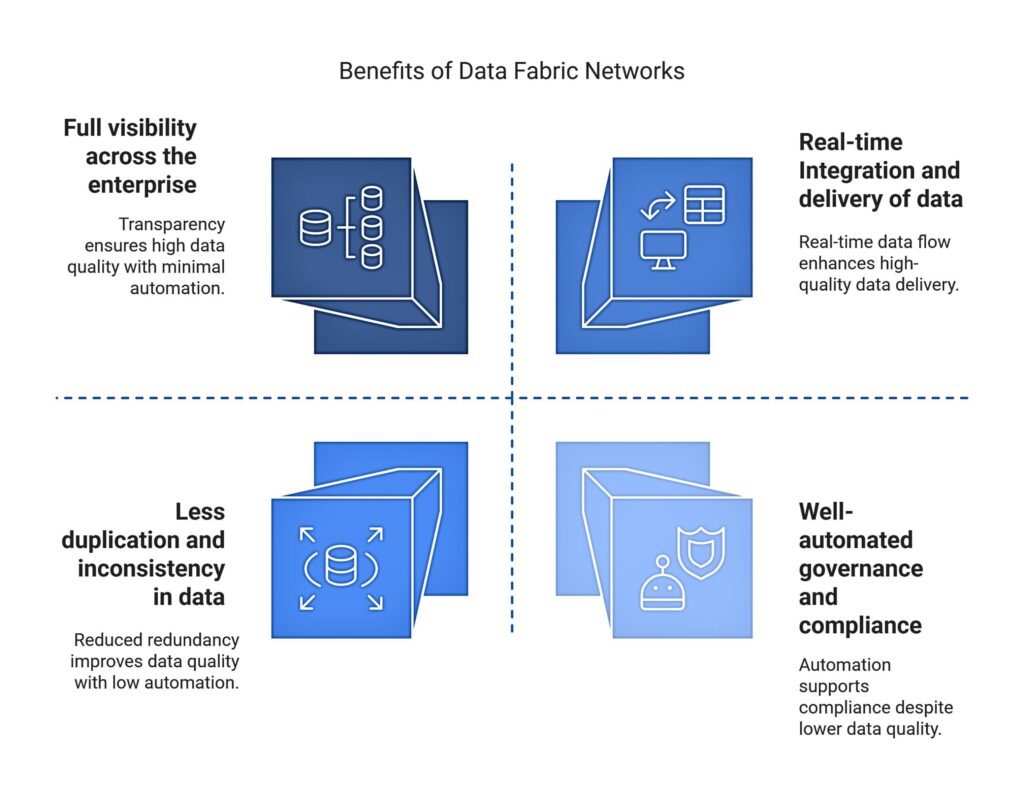
Real-World Use Cases and Examples
When Organizations Opted for Data Mesh:
- Companies in the financial industry that require fast, secure, and domain-controlled access to data.
- Product-led companies scaling microservices and digital offerings.
When They Opted for Data Fabric:
- Healthcare providers uniting EHR, IoT, and analytics.
- Legacy enterprises require seamless access without migration.
When Hybrid Models Made Sense:
- Organizations are bringing Data Fabric for consistency but restricting Mesh to innovation teams or newer business units.
Challenges and Considerations
Complexity in building a Data Mesh from scratch: Very strong domain alignment requires an investment of time, money, and effort to establish decentralized data ownership, governance standards, and culture of data-as-a-product.
Vendor lock-in or platform rigidity with Data Fabric: Relying on a single platform’s ecosystem reaps the benefits for great integration and automation but grows narrow with flexibility in the long-term.
Skills and culture shifts are needed for either: These changes require new roles, new tools, and new processes, most of which will also require training and change management.
Security, privacy and compliance differ: Data Mesh and Data Fabric treat sensitive data differently and thus affect regulatory obligations and internal policy considerations.
Budget issues, time to value, and long-term growth: Both models may require significant initial investment to begin with, but they differ in time to yield measurable ROI and the ability to support future growth.
How to Choose the Right Data Strategy
The choice between Data Mesh and Data Fabric is subject to the organization set-up, objectives, and systems. Data Mesh works efficiently for smaller, independent squads with a focus on experimentation and domain ownership.
Meanwhile, Data Fabric lends itself to organizations that require their information environment to present a unified access pattern to diverse, complex, and often legacy systems. When time to insight is critical and compliance needs tight control, Fabric brings integration and automation.
Data Mesh stands out in agile contexts where empowered teams operate. Here are considerations: Are our teams decentralized and how? Are we driving innovations or getting more streamlined? In terms of tools, Mesh typically consists of Kafka, Snowflake, AWS Lake Formation, and dbt, whereas data Fabric is typically built around Informatica, IBM Cloud Pak, Talend, and Denodo.
Conclusion
Data Mesh vs. Data Fabric is not about which is better overall, but rather, which is better for you specifically. Each has its unique advantages, depending on your size, culture, data maturity, and objectives.
There is no right or wrong answer but understanding the distinction between Data Mesh and Data Fabric would help you in making decisions. Again, pilot projects would clarify those uncertainties. In this day and age of data, it is crucial that your choice of data strategy is aligned with your business model.
Happy Learning!!
Still weighing your options between Mesh and Fabric? Let’s decode it together.
FAQs
What is the main difference between Data Mesh and Data Fabric?
In Data Mesh, data ownership is distributed over domains, while in Data Fabric, intelligent consolidation enables central access to data.
When should a team choose Data Mesh over Data Fabric?
Data Mesh is most appropriate where teams are autonomous, domain-oriented, and have some flexibility in how they manage and scale their data.
Can both strategies be used together?
Yes, many organizations understand Data Fabric as a centralized means to achieve consistency, while applying Mesh principles within specific domains for agility.
Is Data Mesh more suitable for real-time data access?
Data Mesh can generally be accessed real-time within domains, while Fabric usually has better real-time integration across systems.