- May 16, 2025
Did you know the first recorded form of automation dates to ancient Greece, where a steam-powered pigeon by Hero of Alexandria demonstrated early mechanical ingenuity?
For several years, companies would rely heavily on robotic process automation (RPA) to alleviate the burden of manual hours on repetitive tasks.
RPA did deliver measurable efficiency in outcomes, but it also laid bare some clear limitations, a lack of context-awareness, almost no decision-making capability, and extremely brittle performance in the face of any changes.
With the advent of Agentic AI, we can now see the next frontier where systems do not only automate but also think, adapt, and self-act. So, what makes the year 2025 such a crucial year?
Mature AI models, enterprise-ready automation frameworks, and businesses in need of agility are hastening the shift from RPA to Agentic AI. This is not an enhancement but a revolution.
This blog explains the difference between RPA versus Agentic AI, provides real-world use cases of agentic AI, and decodes the significance of this evolution for the future of business automation.
Come, let’s explore this blog together!!
Understanding RPA: The First Wave of Automation

RPA was the first major leap in the intelligent automation evolution. It permitted organizations to automate rule-based repetitive activities like the entry of data, processing of invoices, and generation of reports, thereby enhancing the efficiency of structured predictable workflows.
RPA mimics human action on the UI-level, where every action is based upon a clearly defined set of steps. It thrived in environments where processes were quite stable and required only limited decision-making.
Even though it had early success in the industry, there have been considerable limitations to RPA: it was less agile, not efficient with unstructured data, and often required re-calibration once changes were made to existing systems. These limitations have forced organizations to rethink their automation strategies.
The talk now has moved to RPA vs Agentic AI as the future of business automation involves greater flexibility, intelligence, and scalability. RPA is rules-driven, while Agentic AI transforms into systems that embed context, learn over time, and decide autonomously, all these open new chapters of AI-powered automation.
This evolution is not about the replacement of bots but instead about empowering smarter workflows through next-generation automation tools-and liberating entirely new agentic AI use cases that were previously out of reach.
Introducing Agentic AI: The Next Frontier
One more new entity which narrates task working with respect towards automation in evolving intelligent automation is Agentic AI. Unlike the traditional bots that follow prescribed rules, Agentic AI demonstrates automating decision making, proactive execution, and continuous learning.
So, what does it mean? It means that Agentic AI refers to those agents at AI, which perceive their environment, set goals, and make context-driven decisions, perform actions without human involvement. These agents plan, prioritize, and adapt in addition to reacting to triggers.
The stark difference between RPA and Agentic AI would be as follows:
- RPA Bots – Rule-bound, Reactive, and limited to repetitive, structured processes.
AI agents, in contrast, are goal-directed, capable of dynamic reasoning, and able to coordinate complex workflows autonomously in an ad-hoc manner.
This shift to agentic AI is driven by such technologies as:
- Large Language Models (LLMs) – Understanding and generating instructions in nuanced forms;
- Reinforcement Learning – Teaching agents by trial and feedback what the optimal actions are;
- Vector Databases – Contextual memory and very rapid, intelligent information retrieval.
These next generation automation technologies allow organizations to upgrade from the mere automation of business tasks to strategic enablement.
AI assistants manage customer interactions completely end to end, or autonomous optimization of supply chains in real time, these are real-world agentic AI use cases marking the next chapter towards the future of business automation.
Key Differences Between RPA and Agentic AI
Feature | RPA(Robotic Process Automation) | Agentic AI |
---|---|---|
How it works | Follows fixed rules and scripts | Works toward goals and makes its own decisions |
Data it handles | Only structured, predictable data | Can understand both structured and unstructured data |
Adaptability | Struggles with change or exceptions | Learns and adapts on its own |
Maintenance needs | Needs frequent updates when processes change | Continuously improves with experience |
Decision-making | Cannot make decisions, just follows instructions | Makes smart, context-based decisions |
Learning ability | Does not learn, provides same output every time | Learns from data, feedback, and outcomes |
Integration style | Relies majorly on rigid system APIs | Integrates flexibly across tools using context-aware agents |
Benefits of Moving to Agentic AI
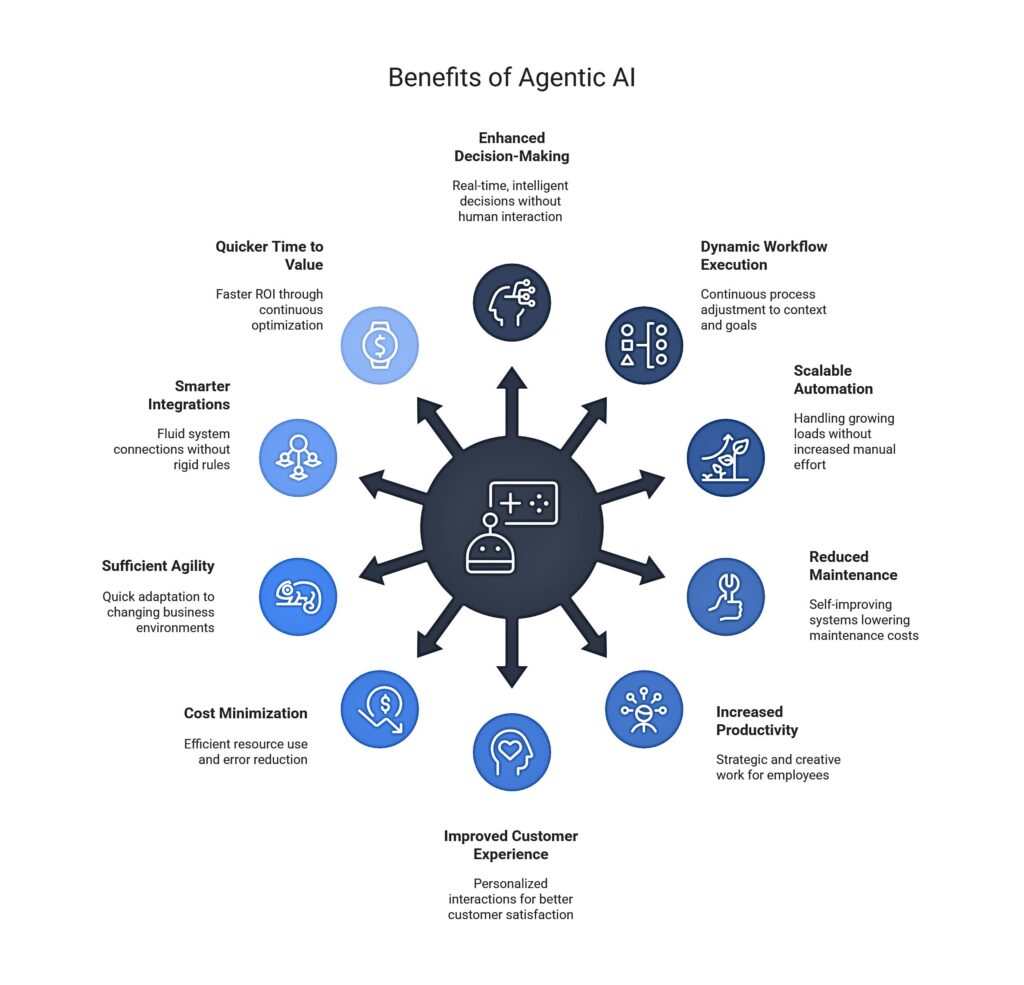
1. Adoption of a Different Decision-Making Mode
Agentic AI enables independent systems to make intelligent decisions in real-time without any human interaction. Therefore, it helps overcome the speed and accuracy gaps in the execution of tasks by human agents.
Most importantly, this revolutionary concept surpasses the limitations of the RPA method in rule-bound execution and forms an important leap in the evolutionary progression of intelligent automation.
2. Dynamic Workflow Execution
Agentic AI runs processes continuously adjusting to context, goals, and input changes as opposed to RPA that has fixed workflows. Thus, it makes automation more responsive and intelligent in executing dynamic enterprise processes.
3. Scalable Automation
Agentic AI takes on growing loads and increasing complexity without proportional increases in manual effort or maintenance. It is for this reason that this form of technology is essential to next-generation tools for automation to make it spread enterprise-wide.
4. Reduced Maintenance
Unlike RPA systems, which need frequent manual modifications and breakdown when there is a change in processes, agentic AI develops through time, learning and adapting along the way. The self-improving nature of these systems allows lowering the costs for maintenance and cutting down the time needed for agentic AI transformation projects.
5. Increase in Employee Productivity
It frees up time for employees to be strategic and creative by taking on repetitive, low-value work. It contributes to making work a satisfying experience and plays a crucial role in the future of business automation.
6. Improved Customer Experience
Agentic AI personalizes customer interaction in real time, incorporating intent, context, and past knowledge in the equation. This results in faster resolutions, richer interactions, and a far better experience than with conventional RPA systems.
7. Cost Minimization
Agentic intelligence contributes to reduced operational costs through maximum use of resources and minimization of errors and downtimes. A smarter approach of automation brings business an even better outcome at a lower total cost.
8. Sufficient Agility
Agentic AI can allow organizations to modify processes, decisions, and priorities in changing business environments on-the-fly. That agility is the competitive edge and is really a hallmark of AI-powered automation platforms.
9. Smarter Integrations
Context aware agents can understand, connect, and act across a variety of systems without the rigidity of predefined API rules. This makes integration more fluid inside agentic AI applications, greasing friction and increasing efficiency.
10. Quicker Time to Value
Quick results are delivered by agentic AI due to lesser setup requirements, manual interventions, and continuous optimization through learning. Thus, faster ROI accrues, fortifying the organization’s position in the intelligent automation evolutionary landscape.
Real-World Use Cases of Agentic AI in 2025
- Customer Support: It is a library automation process that will resolve tickets on its own.
Agentic AI has the power to revolutionize customer support by solving tickets independently. These AI agents analyze the problem, find viable solutions, and answer customers in real-time, reducing the response time drastically and increasing customer satisfaction.
- Finance: AI Agents Reconciling Transactions Across Systems
Agentic AI agents automate the complex reconciling transactions across various systems in finance. They also check for discrepancies, match records, and flag anomalies independently, avoiding human errors and speeding up financial operations.
- Healthcare: Patient Record Coordination Across Providers
Agentic AI enables coordination of patient records across more providers and systems to streamline healthcare operations. These AI agents can handle data-sharing so that updated patient information can be accessible to providers, patient treatment improvements, and decreased administrative burdens.
- IT Ops: Self-healing Infrastructure Bots
In IT operations, agentic AI powers self-healing infrastructure bots that can detect, diagnose, and resolve issues as they appear before they lapse into performance impact. These autonomic systems optimize uptime besides reducing need for human intervention, thereby ensuring neat operations with minimal disruptions.
- Sales: AI Assistants Performing Lead Research and Outreach
In sales, agentic AI does lead research and outreach autonomously. It provides real-time analysis of customer data and behavior patterns using its powerful algorithms to pinpoint high-value leads, initiate contact, and nurture, thus allowing a team to concentrate on closing deals.
When to Choose RPA vs Agentic AI
Best-fit Scenarios for RPA:
Rule-based, repetitive tasks serve best for RPA-like data entry, report generation, or simple customer service. Structured processes with a high degree of predictability and less decision-making are apt for RPA.
Best-fit Scenarios for Agentic AI:
Agentic AI is well-off to solve complex tasks that are dynamic in nature and involve multiple systems with real-time decision-making. Notable examples include customer support, data reconciliation between systems, or process optimization across departments.
Hybrid Approach in Modern Enterprises:
Many enterprises work best with a hybrid approach, incorporating RPA for the mundane tasks and Agentic AI for the more convoluted and adaptive workflows. This balance provides optimal cost-benefit and performance beyond the RPA constraints.
Conclusion
The move from RPA to Agentic AI is a true revolution in business automation. RPA is still the best oracle at executing generic and repetitive and mundane tasks, while Agentic AI provides a much lively and more vibrant, intelligent-sounding interpretation of autonomy and decision-making in overly complex processes.
Knowing these distinctions and the corresponding benefits informs businesses about the right automation choice in relation to their needs, tech maturity, and complexity of processes. This is the evolution of the futuristic scenario-Revolution through RPA, Agentic AI, or a combination of both as a family-future enabling organizations to redefine their process efficiencies, increase productivity, and stay ahead of the curve in an increasingly automated world.
Happy Learning!!
Not sure whether RPA or Agentic AI fits your needs?
Our experts can help you decide.
FAQs
What are the key differences between RPA and Agentic AI in intelligent automation?
RPA is rule-based and handles repetitive, structured tasks with a little adaptability or learning. Agentic AI, on the other hand, relies on autonomous, goal-driven logic, which allows it to deal with dynamic workflows, unstructured data, and continuous learning. This implies a huge paradigm shift in the evolution of intelligent automation.
How can businesses transition from traditional RPA to Agentic AI systems?
Organizations should start looking for complex cross-functional processes that are simply too big for RPA and pilot Agentic AI use cases. A hybrid approach using RPA on simpler jobs and Agentic AI on workflows requiring more adaptability lends itself to an easier transition with scalable gains.
What are the benefits of adopting Agentic AI for enterprise automation in 2025?
Agentic AI means autonomous decision-making, intelligent integration, and self-correcting workflows, which dramatically increase productivity and agility. It will also bring along cost savings, swifter time-to-value, and a cutting edge in business automation in the long term.
Are there any risks or challenges associated with implementing Agentic AI?
Challenges like data governance, integration complexities, and trust in autonomous systems. These risks, however, can be tamed with requisite planning and structure to unleash the untapped potential of AI-powered automation.